April 25th, 2025 / News
Q&A with John Waters, Director of Revenue Cycle Product at CliniComp How AI Is Automating—and Improving—Revenue Cycle Management
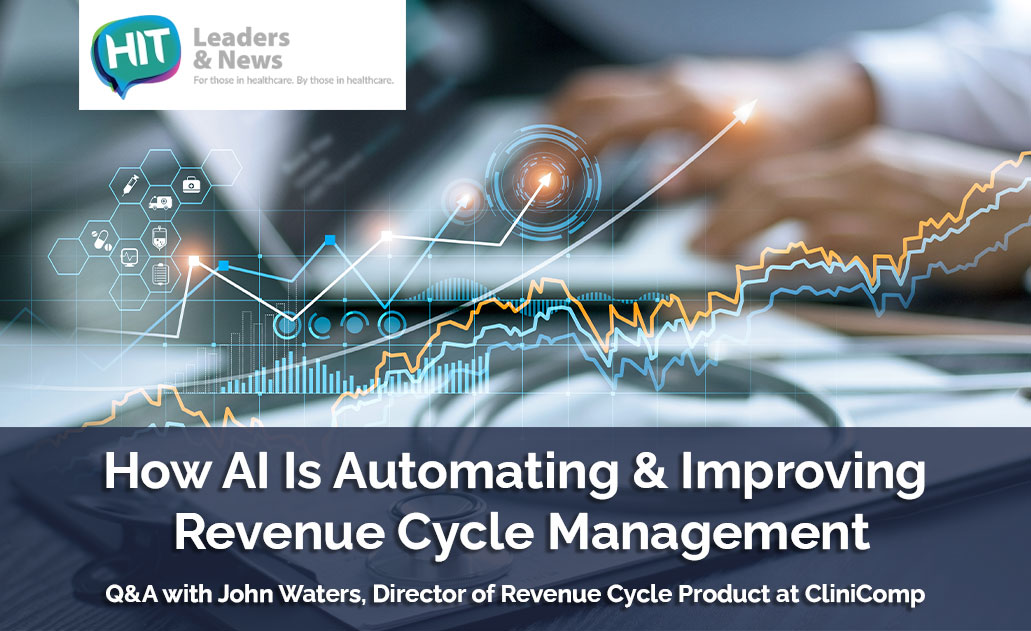
For decades, revenue cycle management (RCM) has been one of the most labor-intensive and error-prone functions in healthcare operations. From prior authorizations to claim denials, coding compliance to eligibility verification, inefficiencies in the RCM workflow have long drained staff resources and delayed revenue.
But with the emergence of AI-powered automation and predictive analytics, a new era of intelligent RCM is taking shape. In this exclusive HIT Leaders & News Q&A, John Waters, Director of Revenue Cycle Product at CliniComp, shares how artificial intelligence is transforming this traditionally manual space. Waters discusses how machine learning can reduce denials, boost coding accuracy, and improve authorization workflows—while also calling out the real-world barriers that health systems must address to fully unlock AI’s potential in the revenue cycle.
How do you see AI transforming the traditional RCM workflow, and what specific inefficiencies do you think artificial intelligence can address most effectively?
Historically, revenue cycle is an area that is extremely manual and time/labor-intensive. That is why there is a lot of buzz around artificial intelligence in the revenue cycle management (RCM) space. There are rules and guidelines around everything that we do in RCM. When we can teach AI the various rules and regulations, the system can automate a lot of the tedious tasks for revenue cycle end users. AI assistance can also mitigate unnecessary rework by doing things like checking insurance claims before they go out for accuracy and completeness which reduces the likelihood of denials. When AI works to compliment an EHR and offset tasks and errors in RCM, it can do wonders.
What are some of the challenges that healthcare organizations face when implementing AI-driven coding assistance, and how can they be mitigated?
Just like claims submissions, there are guidelines and regulations to follow on how a medical visit is coded, and medical coders are held to standards for accuracy. While complex, the logic around coding makes it possible for AI to understand and process. One of the challenges, and this is not unique to AI assistance, is that the coding is only as precise as the medical visit documentation. If your organization experiences issues with inaccurate, incomplete, missing or late documentation – that is going to reduce how effective AI can be. On the other hand, AI can help ensure completion of coding and claims before claims leave for payment. Many hospitals have auditors or auditing department staff that work to correct issues with documentation. That is also a labor-intensive process. This is another role for AI assistance – to find and flag inaccuracies and gaps in documentation so they can be addressed earlier.
In what ways can AI improve denial management, and how does it compare to traditional manual approaches?
The nice thing about AI is that it is intelligent enough to know how much confidence should be placed in specific answers it provides. That is really, really important.
One of the hot topics in RCM now is which tasks are best suited for AI assistance throughout the authorization, documentation, coding, claims and payment processes. One goal is to minimize denials on the back end.
Therefore, it is important to avoid denials in the first place, and without AI assistance denied claims can take weeks to clean up and resubmit.
Another role for AI is identifying denial patterns and suggesting ways to avoid them in the future. If a particular payer repeatedly denies certain claims, AI can detect that trend over time. When AI identifies what is causing denials, then it is reasonable to assume that you could avoid those denials by changing your processes, or by using AI earlier in the process to automate or modify submissions.
How can machine learning models be trained to predict and prevent claim denials before billing even begins?
It all starts with data, and, in general, the more data used to train the machine learning system, the better. The system should have enough information to understand why an insurer is paying versus not paying a claim. The system needs to understand how billing works within RCM. Armed with accurate and sufficient training data, a machine learning system can identify why specific insurances are issuing denials and suggest ways to change processes to minimize the risk for denials going forward.
What role does AI play in ensuring coding accuracy before claims are submitted, and how can it help reduce the risk of compliance issues?
Like coding and claims, there are rules and regulations around compliance that AI can learn and interpret to save time. Again, AI can flag items that are inaccurate or not aligned with the compliance regulations and this process can be automated. Historically, auditors would perform spot checks to ensure processes were meeting compliance regulations. Auditors that use AI assistance to verify compliance can speed up their processes and boost accuracy. In general, AI is able to help automate processes from the most simple claims to the most complex; however, organizations may prefer to more heavily involve end users in more complex cases to ensure accuracy and adherence to regulations, policies, etc.
How can AI be leveraged to streamline authorization and eligibility verification, and what impact does this have on revenue cycle efficiency?
This is a huge topic in RCM because authorization-related billing problems are oftentimes one of the main problems that ultimately delay cash. It is not a new challenge – and there are multiple rules and regulations among different insurance companies. It can be complicated to get it right the first time. Prior authorizations are often very complex – they are based on a spider web of payer-specific rules and regulations. That is why people are talking about adding AI in these areas. AI can not only check verifications and authorizations to make sure the requested information is complete, but the technology can also spot patterns and automate processes moving forward. When AI assistance identifies issues sooner, the entire process becomes more efficient. Lastly, when AI can help to inform the system when an authorization is required and how it should be documented, the likelihood of wasted effort is minimized.
What are the biggest barriers, barriers to AI adoption and RCM, and how can organizations overcome these challenges to maximize the benefits?
There are multiple challenges. You need a lot of data to train the system, and AI is only as good as the training data it receives. For RCM optimization, I would avoid AI solutions whose expertise is not in the healthcare space. Seek out technologies that are integrated and natively embedded to streamline current system processes.